The media landscape is undergoing a significant transformation, thanks to advancements in Artificial Intelligence (AI). This shift is presenting media companies, including the BBC, with new capabilities and opportunities to enhance their services and content delivery. From adding subtitles to programs on BBC Sounds to translating content into multiple languages on BBC News, AI is already making a positive impact. The BBC is also developing AI tools to assist staff in daily tasks and exploring innovative ways to provide audiences with new experiences, such as personal tutors on Bitesize.
However, with great power comes great responsibility. While AI promises substantial value, it also poses significant challenges for audiences and the UK’s information ecosystem. One key concern is the role of AI assistants, like those from OpenAI, Google, and Microsoft, in distorting journalism.
The Research on AI Assistants and News Accuracy
AI assistants are being adapted to perform various tasks, including drafting emails, analyzing data, and summarizing information. They also provide answers to questions about news and current affairs, often repurposing content from publishers’ websites without permission (Economic Times, 2024). To understand the accuracy of news-related outputs from AI assistants, the BBC conducted research on four prominent AI assistants: OpenAI’s ChatGPT, Microsoft’s Copilot, Google’s Gemini, and Perplexity.
During the research, the AI assistants were given access to the BBC website and asked questions about the news, with the prompt to use BBC News articles as sources where possible. BBC journalists, experts in the topics, reviewed the AI-generated answers based on criteria such as accuracy, impartiality, and representation of BBC content.
Findings and Implications
The research revealed alarming results. AI assistants produced answers containing significant inaccuracies and distorted BBC content:
- 51% of AI answers to news questions had significant issues.
- 19% of AI answers citing BBC content contained factual errors.
- 13% of quotes from BBC articles were either altered or not present in the cited article.
These inaccuracies matter because they undermine trust in the news, essential for a society that relies on a shared understanding of facts. Inaccuracies from AI assistants can be easily amplified when shared on social networks, leading to real harm. News publishers must ensure their content is used accurately and with permission. Internal research shows that when AI assistants cite trusted brands like the BBC, audiences are more likely to trust the answer, even if it is incorrect.
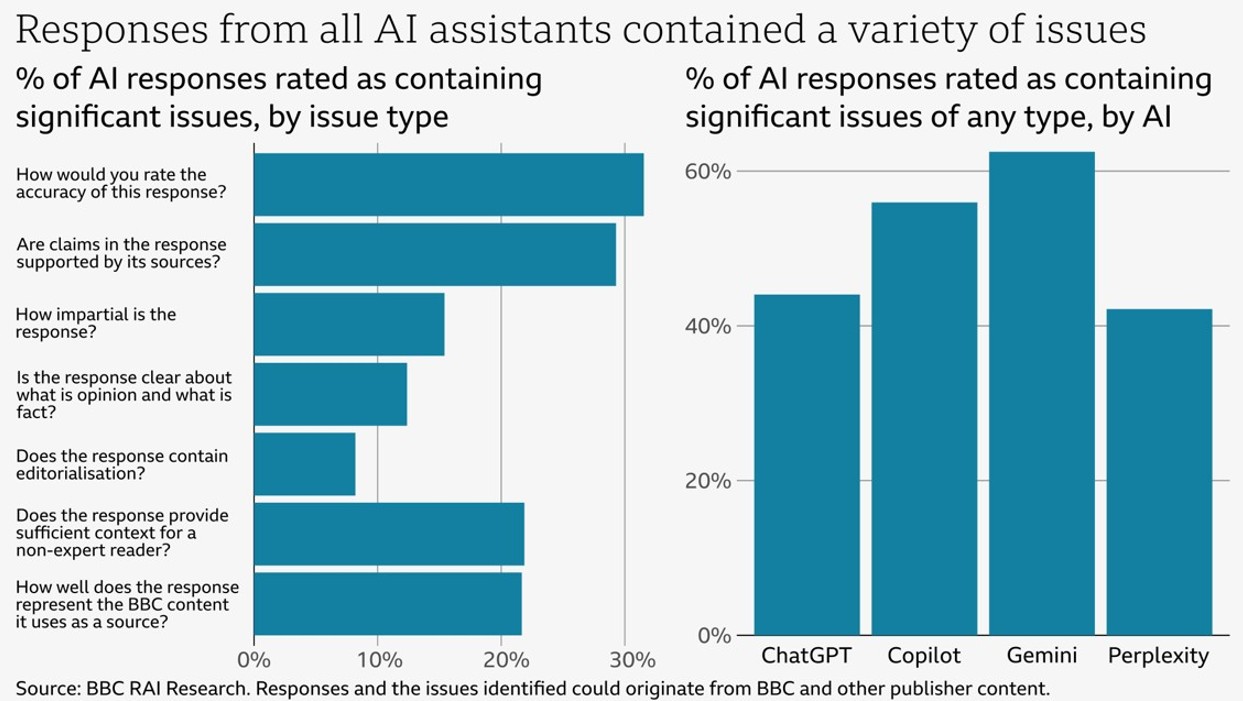
Examples of Distortion
Individual errors from AI assistants highlight broader issues. For instance, Google’s Gemini incorrectly stated that “The NHS advises people not to start vaping,” while the NHS actually recommends vaping as a method to quit smoking. Microsoft’s Copilot incorrectly reported details about Gisèle Pelicot’s discovery of crimes against her, and Perplexity misstated the date of Michael Mosley’s death and misquoted a statement from Liam Payne’s family. OpenAI’s ChatGPT falsely claimed in December 2024 that Ismail Haniyeh, assassinated in Iran in July 2024, was part of Hamas leadership.
The Unknown Scale of the Issue
The scope and scale of errors and content distortion by AI assistants remain unknown. AI assistants provide answers on a wide range of questions, and users can receive different answers to the same question. The extent of the issue is unclear to audiences, media companies, regulators, and possibly even AI companies.
Urgent Need for Accurate and Trustworthy AI
AI assistants currently cannot be relied upon to provide accurate news and risk misleading audiences. Unlike professional news outlets that correct errors, AI applications lack mechanisms for error correction. This issue may extend to other areas where reliability and accuracy are crucial, such as health, education, and security.
Next Steps and Call to Action
As the use of AI assistants grows, it is critical to ensure they provide accurate and trustworthy information. Publishers, like the BBC, should control how their content is used, and AI companies should transparently show how assistants process news and the scale of errors.
The proposed steps to Avoid Distortions and Inaccuracies by AI Assistants:
- Content Usage Policies: Develop clear policies on how AI assistants can use and repurpose content from publishers.
- Improvement in AI Training: Work together to improve AI models by incorporating feedback from different organizations to reduce distortions.
- Public Disclosures: AI companies should disclose how their systems process and summarize news content.
- Error Reporting Mechanism: Implement systems for users to report errors and inaccuracies in AI-generated content.
- Regulations on AI Content Use: Develop regulations that ensure AI assistants use content from news publishers accurately and with permission.
- Regular Audits: Conduct regular audits of AI systems to check for compliance with accuracy and ethical standards.
- Improved Algorithms: Invest in advanced algorithms that better understand and accurately summarize content.
- Real-time Monitoring: Implement real-time monitoring systems to detect and correct inaccuracies in AI responses.
- Human-in-the-Loop Systems: Integrate human oversight in AI systems to review and approve content summaries, especially for sensitive topics.
- Feedback Mechanisms: Collect feedback from practitioners, experts, researchers, journalists, and other relevant communities to continuously improve AI systems.
- Educational Initiatives: Increase AI literacy among audiences to help them critically evaluate AI-generated content.
- Bias Mitigation: Implement strategies to mitigate bias in AI systems to ensure balanced and fair reporting.
- Regular Updates: Update AI models regularly with the latest information and improved processing techniques.
Independent Evaluations: Conduct independent evaluations of AI systems to identify and rectify potential flaws.
Reference: Representation of BBC News content in AI Assistants